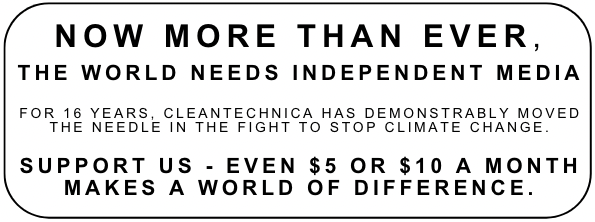
Sign up for daily news updates from CleanTechnica on email. Or follow us on Google News!
Deciding where to build a solar or wind farm? MIT engineers show how detailed mapping of weather conditions and energy demand can guide optimization for siting renewable energy installations.
Prior to now, there were few integrated sources for combining data from individual developers or utilities, which made it more guesswork to choose and plan renewable energy sites efficiently. MIT engineers recently changed that. They demonstrate how precise mapping of energy consumption and weather patterns may direct the placement of renewable energy installations with high efficiency.
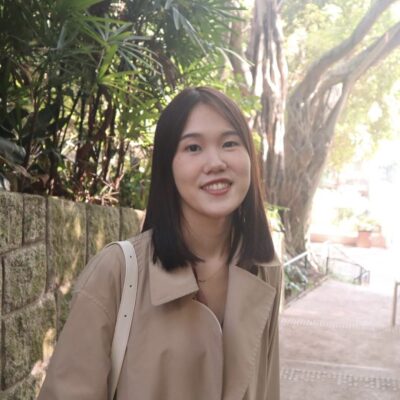
Recent research demonstrates that the design of renewable power plants can be significantly impacted by regional-level planning that makes use of fine-grained weather data, energy demand data, and energy system modeling. Additionally, this results in operations that are more lucrative and efficient.
Liying Qiu, the lead author of the recent study in the journal Cell Reports Sustainability, explains that with her team’s new approach, “we can harness the resource complementarity, which means that renewable resources of different types, such as wind and solar, or different locations, can compensate for each other in time and space. This potential for spatial complementarity to improve system design has not been emphasized and quantified in existing large-scale planning.”
“We are actually trying to use the natural variability itself to address the variability,” she explains. “Such complementarity will become ever more important as variable renewable energy sources account for a greater proportion of power entering the grid,” she says. The idea is to “coordinate the peaks and valleys of production and demand more smoothly.”
Reading through her work, it appears to be a comprehensive equilibrium of weather and energy with a high level of thought and investigation.
Typically, in planning large-scale renewable energy installations, Qiu says it’s been loose and broad-brushed — “some work on a country level, for example, saying that 30 percent of energy should be wind and 20 percent solar. That’s very general.”
For this study, the team analyzed both weather data and energy system modeling at a spatial scale of less than 10 kilometers (approximately 6 miles).
“It’s a way of determining where we should exactly build each renewable energy plant, rather than just saying this city should have this many wind or solar farms,” she interprets.
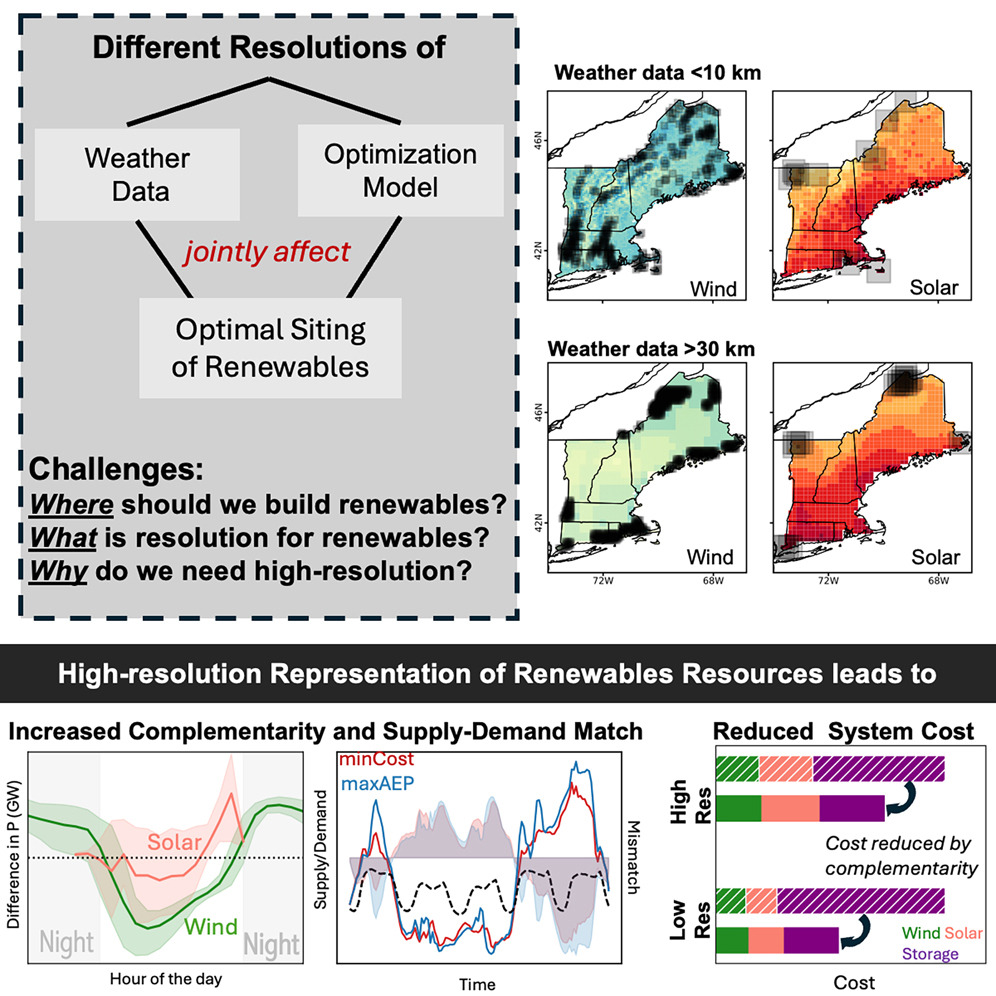
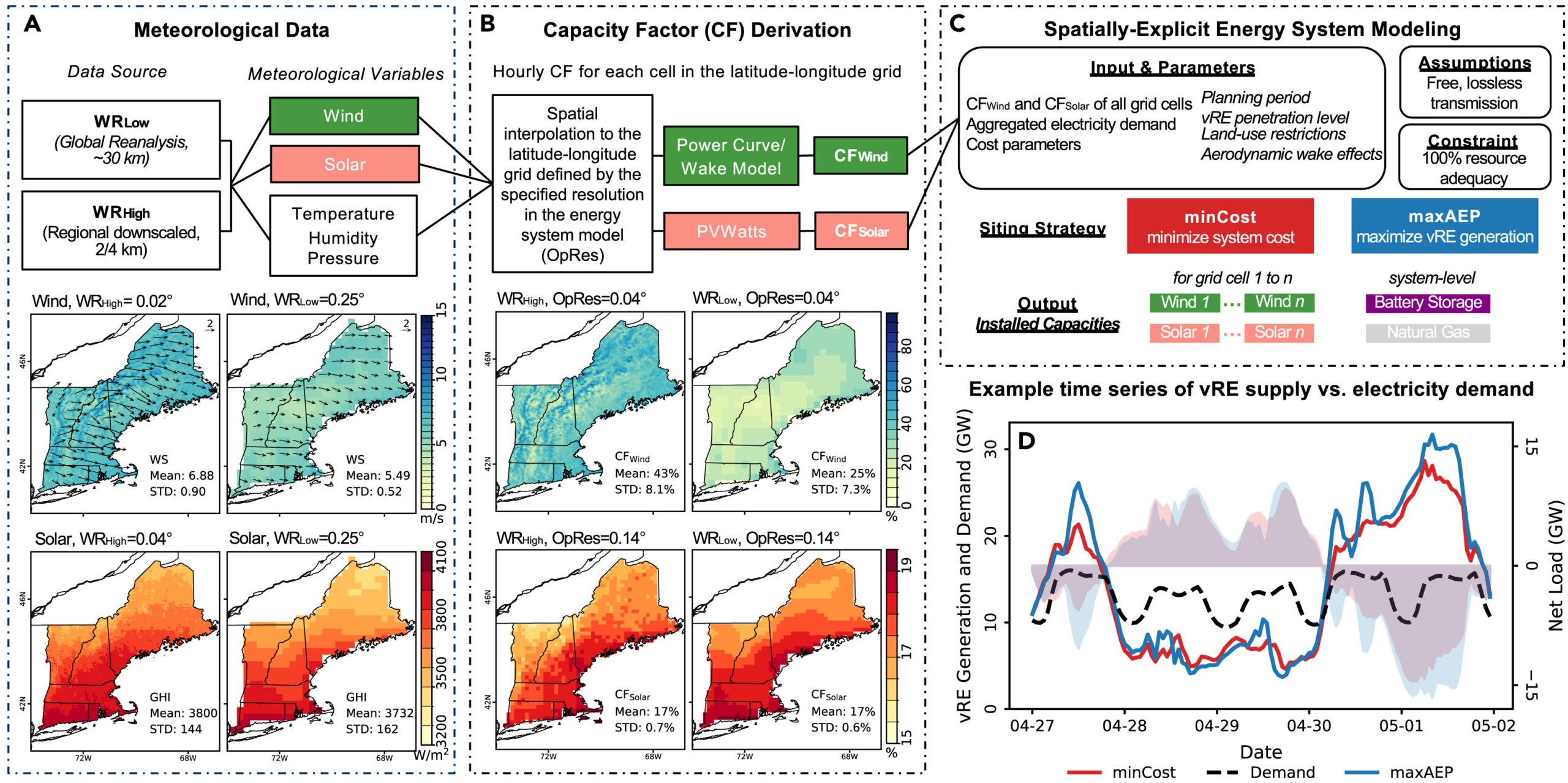
In order to maximize the use of renewable resources, the results demonstrate the advantages of coordinating the site of solar farms, wind farms, and storage systems while accounting for local and temporal fluctuations in wind, sunshine, and energy demand. The researchers discovered that this strategy can maximize the availability of clean power when needed while minimizing the requirement for significant storage investments and, consequently, the overall system cost.
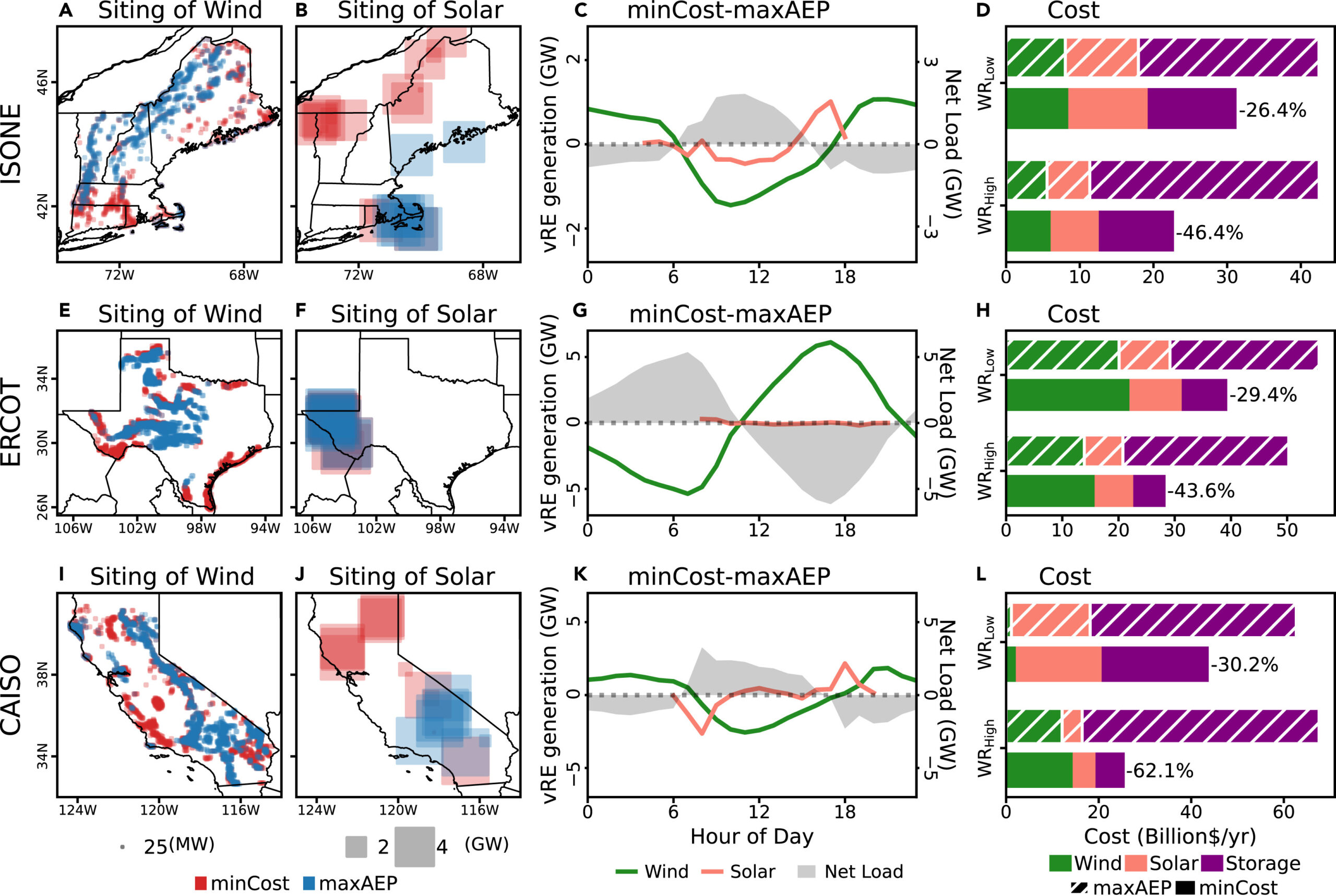
“The study, which will appear in the journal Cell Reports Sustainability, was co-authored by Liying Qiu and Rahman Khorramfar, postdocs in MIT’s Department of Civil and Environmental Engineering, and professors Saurabh Amin and Michael Howland.”
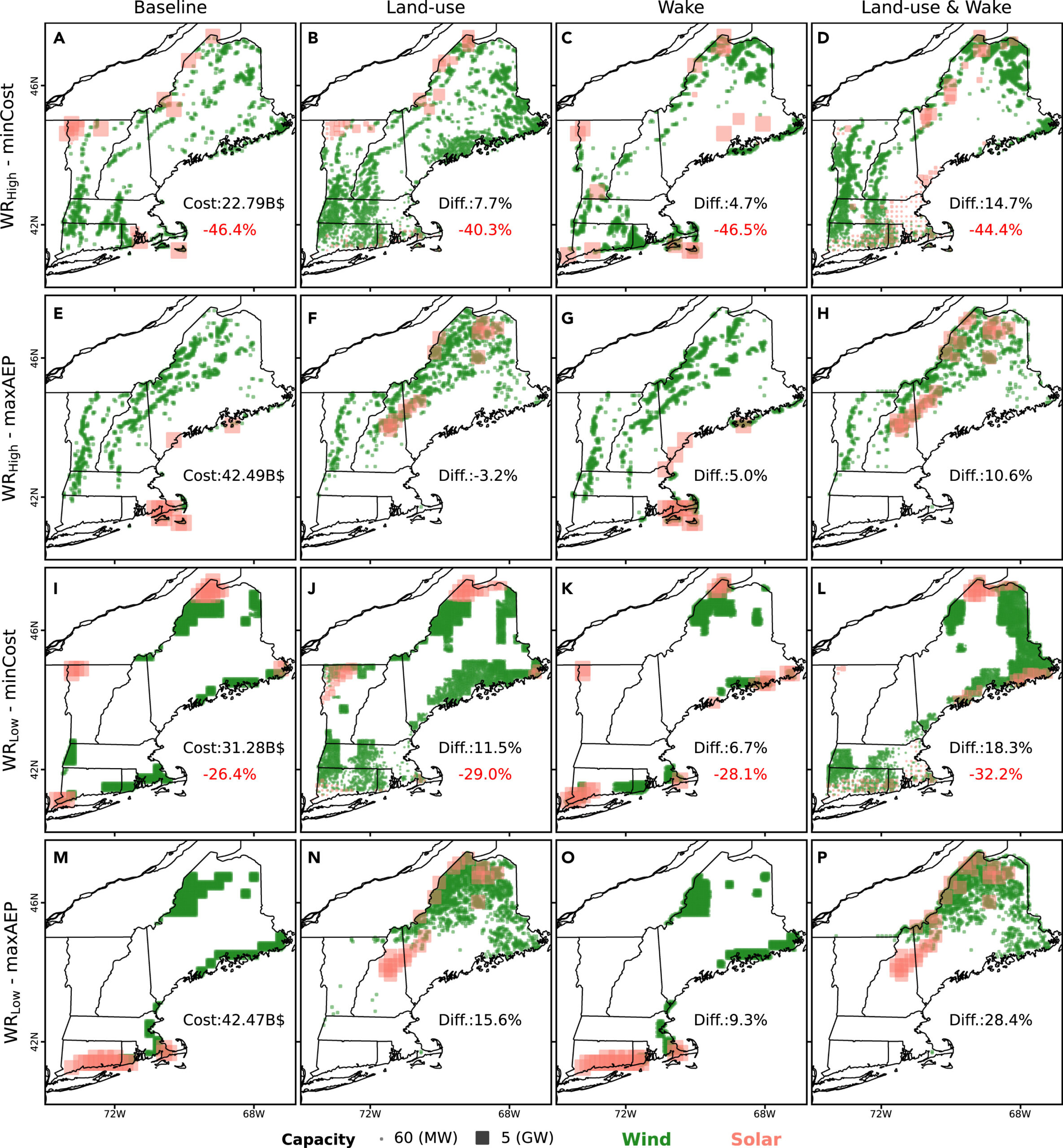
To assemble their data and enable high-resolution planning, the researchers used a number of until now unintegrated sources. They employed high-resolution meteorological data from the National Renewable Energy Laboratory, which is publicly available at 2-kilometer resolution but is rarely used in a planning model of this fine scale.
“These data were combined with an energy system model they developed to optimize siting at a sub-10-kilometer resolution. To get a sense of how the fine-scale data and model made a difference in different regions, they focused on three U.S. regions — New England, Texas, and California — analyzing up to 138,271 possible siting locations simultaneously for a single region.”
By comparing the results of siting based on a typical method vs. their high-resolution approach, the team showed that “resource complementarity really helps us reduce the system cost by aligning renewable power generation with demand,” which should translate directly to real-world decision-making, Qiu says. “If an individual developer wants to build a wind or solar farm and just goes to where there is the most wind or solar resource on average, it may not necessarily guarantee the best fit into a decarbonized energy systems.”
Power supply and consumption fluctuate hourly and monthly as the seasons change. “What we are trying to do is minimize the difference between the energy supply and demand rather than simply supplying as much renewable energy as possible,” Qiu says. “Sometimes your generation cannot be utilized by the system, while at other times, you don’t have enough to match the demand.”
Rahman Khorramfar, also a postdoc in MIT’s Department of Civil and Environmental Engineering, says that this work “highlights the importance of data-driven decision making in energy planning.” The work shows that using such high-resolution data coupled with a carefully formulated energy planning model “can drive the system cost down, and ultimately offer more cost-effective pathways for energy transition.”
According to the researchers, its framework is extremely adaptable to anyplace, accounting for local geophysical and other factors. Peak west winds in Texas, for example, come in the morning, but they occur in the afternoon on the south coast, so the two naturally enhance each other.
In New England, for instance, the new research indicates that more wind farms should be built in areas with a good wind resource at night, when solar energy is lacking. Some areas are windier at night, while others have more wind during the day.
Surprising Data: Significant Gains Results in Less Need for Energy Storage
“One thing that was surprising about the findings, says Amin, who is a principal investigator in the Laboratory of Information and Data Systems, is how significant the gains were from analyzing relatively short-term variations in inputs and outputs that take place in a 24-hour period. “The kind of cost-saving potential by trying to harness complexity within a day was not something that one would have expected before this study,” he says.
In addition, Amin says, it was also surprising how much this kind of modeling could reduce the need for storage as part of these energy systems. “This study shows that there is actually a hidden cost-saving potential in exploiting local patterns in weather that can result in a monetary reduction in storage cost.”
The system-level analysis and planning suggested by this study, Howland says, “changes how we think about where we site renewable power plants and how we design those renewable plants so that they maximally serve the energy grid. It has to go beyond just driving down the cost of energy of individual wind or solar farms. And these new insights can only be realized if we continue collaborating across traditional research boundaries by integrating expertise in fluid dynamics, atmospheric science, and energy engineering.”

Source: MIT News, David L. Chandler
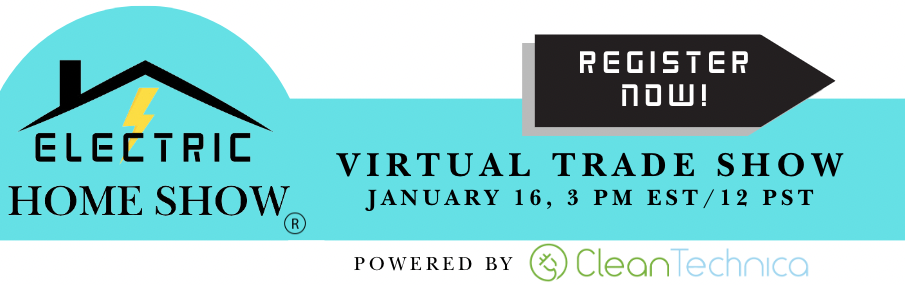
Chip in a few dollars a month to help support independent cleantech coverage that helps to accelerate the cleantech revolution!
Have a tip for CleanTechnica? Want to advertise? Want to suggest a guest for our CleanTech Talk podcast? Contact us here.
Sign up for our daily newsletter for 15 new cleantech stories a day. Or sign up for our weekly one if daily is too frequent.
CleanTechnica uses affiliate links. See our policy here.
CleanTechnica’s Comment Policy